What is Machine Learning in Medicine - Global Market?
Machine learning in medicine is a rapidly evolving field that leverages advanced algorithms and computational power to analyze vast amounts of medical data, leading to improved patient outcomes and more efficient healthcare systems. This global market is driven by the increasing availability of healthcare data and the need for personalized medicine. Machine learning algorithms can identify patterns and insights from complex datasets, such as electronic health records, medical imaging, and genomic data, which are often too intricate for traditional analytical methods. These insights can assist in early disease detection, prognosis prediction, and treatment optimization. The integration of machine learning in medicine also supports the development of innovative diagnostic tools and therapeutic strategies, enhancing the precision and accuracy of medical interventions. As healthcare systems worldwide strive to improve patient care while managing costs, the adoption of machine learning technologies is expected to grow, offering significant opportunities for innovation and advancement in the medical field. The global market for machine learning in medicine is poised for substantial growth, driven by technological advancements, increased investment in healthcare IT, and the rising demand for data-driven decision-making in clinical practice.
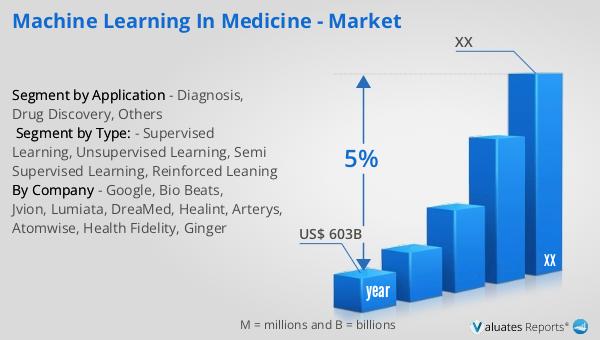
Supervised Learning, Unsupervised Learning, Semi Supervised Learning, Reinforced Leaning in the Machine Learning in Medicine - Global Market:
Supervised learning, unsupervised learning, semi-supervised learning, and reinforcement learning are key machine learning paradigms that play crucial roles in the medical field. Supervised learning involves training algorithms on labeled datasets, where the input data is paired with the correct output. This approach is particularly useful in medical diagnostics, where algorithms can be trained to recognize patterns in medical images or predict disease outcomes based on historical patient data. For instance, supervised learning models can be used to identify tumors in radiology images or predict patient responses to specific treatments. Unsupervised learning, on the other hand, deals with unlabeled data and is used to uncover hidden patterns or groupings within the data. In medicine, unsupervised learning can help in clustering patients with similar symptoms or genetic profiles, which can lead to more personalized treatment plans. It can also be used to identify novel disease subtypes or predict disease progression. Semi-supervised learning combines elements of both supervised and unsupervised learning, utilizing a small amount of labeled data alongside a larger pool of unlabeled data. This approach is beneficial in medical research where labeled data is scarce or expensive to obtain. Semi-supervised learning can enhance the accuracy of predictive models by leveraging the vast amounts of unlabeled data available in healthcare. Reinforcement learning is a type of machine learning where algorithms learn to make decisions by receiving feedback from their actions in a dynamic environment. In medicine, reinforcement learning can be applied to optimize treatment strategies, such as determining the best sequence of therapies for cancer patients or managing chronic diseases like diabetes. By continuously learning from patient responses, reinforcement learning models can adapt and improve over time, leading to more effective and personalized care. Each of these learning paradigms offers unique advantages and can be applied to various aspects of medical practice, from diagnostics and treatment planning to drug discovery and patient management. As the global market for machine learning in medicine continues to expand, these techniques will play an increasingly important role in transforming healthcare delivery and improving patient outcomes.
Diagnosis, Drug Discovery, Others in the Machine Learning in Medicine - Global Market:
Machine learning is revolutionizing the field of medicine by enhancing the accuracy and efficiency of diagnosis, accelerating drug discovery, and improving various other aspects of healthcare. In the realm of diagnosis, machine learning algorithms are being used to analyze medical images, such as X-rays, MRIs, and CT scans, to detect abnormalities and diseases with high precision. These algorithms can identify patterns and features that may be missed by human eyes, leading to earlier and more accurate diagnoses. For example, machine learning models have been developed to detect breast cancer in mammograms, identify diabetic retinopathy in retinal images, and diagnose skin cancer from dermatological images. In drug discovery, machine learning is being used to analyze large datasets of chemical compounds and biological data to identify potential drug candidates. By predicting the interactions between drugs and their targets, machine learning can significantly reduce the time and cost associated with traditional drug discovery processes. This technology is also being used to repurpose existing drugs for new therapeutic uses, offering new treatment options for patients. Beyond diagnosis and drug discovery, machine learning is being applied to various other areas of medicine, such as personalized medicine, patient monitoring, and healthcare management. In personalized medicine, machine learning algorithms analyze genetic, environmental, and lifestyle data to tailor treatments to individual patients, improving the efficacy and safety of therapies. In patient monitoring, machine learning models can predict patient deterioration or adverse events by analyzing real-time data from wearable devices and electronic health records. This enables proactive interventions and improves patient outcomes. In healthcare management, machine learning is being used to optimize resource allocation, streamline administrative processes, and enhance decision-making. By analyzing data on patient flow, hospital operations, and treatment outcomes, machine learning can help healthcare providers improve efficiency and reduce costs. As the global market for machine learning in medicine continues to grow, these applications are expected to become increasingly integral to healthcare delivery, driving innovation and improving patient care worldwide.
Machine Learning in Medicine - Global Market Outlook:
Based on our analysis, the worldwide market for medical devices is projected to reach approximately $603 billion in 2023, with an anticipated compound annual growth rate (CAGR) of 5% over the next six years. This growth is driven by several factors, including technological advancements, an aging global population, and the increasing prevalence of chronic diseases. As healthcare systems around the world strive to improve patient outcomes and manage costs, the demand for innovative medical devices is expected to rise. These devices, which range from diagnostic tools and monitoring equipment to therapeutic and surgical instruments, play a crucial role in modern healthcare by enabling early disease detection, accurate diagnosis, and effective treatment. The integration of machine learning and artificial intelligence into medical devices is also contributing to market growth, as these technologies enhance the capabilities and efficiency of medical devices. For instance, AI-powered diagnostic tools can analyze medical images with high precision, while machine learning algorithms can predict patient outcomes and optimize treatment plans. Additionally, the increasing adoption of telemedicine and remote patient monitoring is driving demand for connected medical devices that facilitate virtual care and continuous health monitoring. As the global market for medical devices continues to expand, manufacturers and healthcare providers are expected to invest in research and development to create innovative solutions that address the evolving needs of patients and healthcare systems. This growth presents significant opportunities for companies operating in the medical device industry, as well as for investors seeking to capitalize on the expanding healthcare market.
Report Metric | Details |
Report Name | Machine Learning in Medicine - Market |
Accounted market size in year | US$ 603 billion |
CAGR | 5% |
Base Year | year |
Segment by Type: |
|
Segment by Application |
|
By Region |
|
By Company | Google, Bio Beats, Jvion, Lumiata, DreaMed, Healint, Arterys, Atomwise, Health Fidelity, Ginger |
Forecast units | USD million in value |
Report coverage | Revenue and volume forecast, company share, competitive landscape, growth factors and trends |